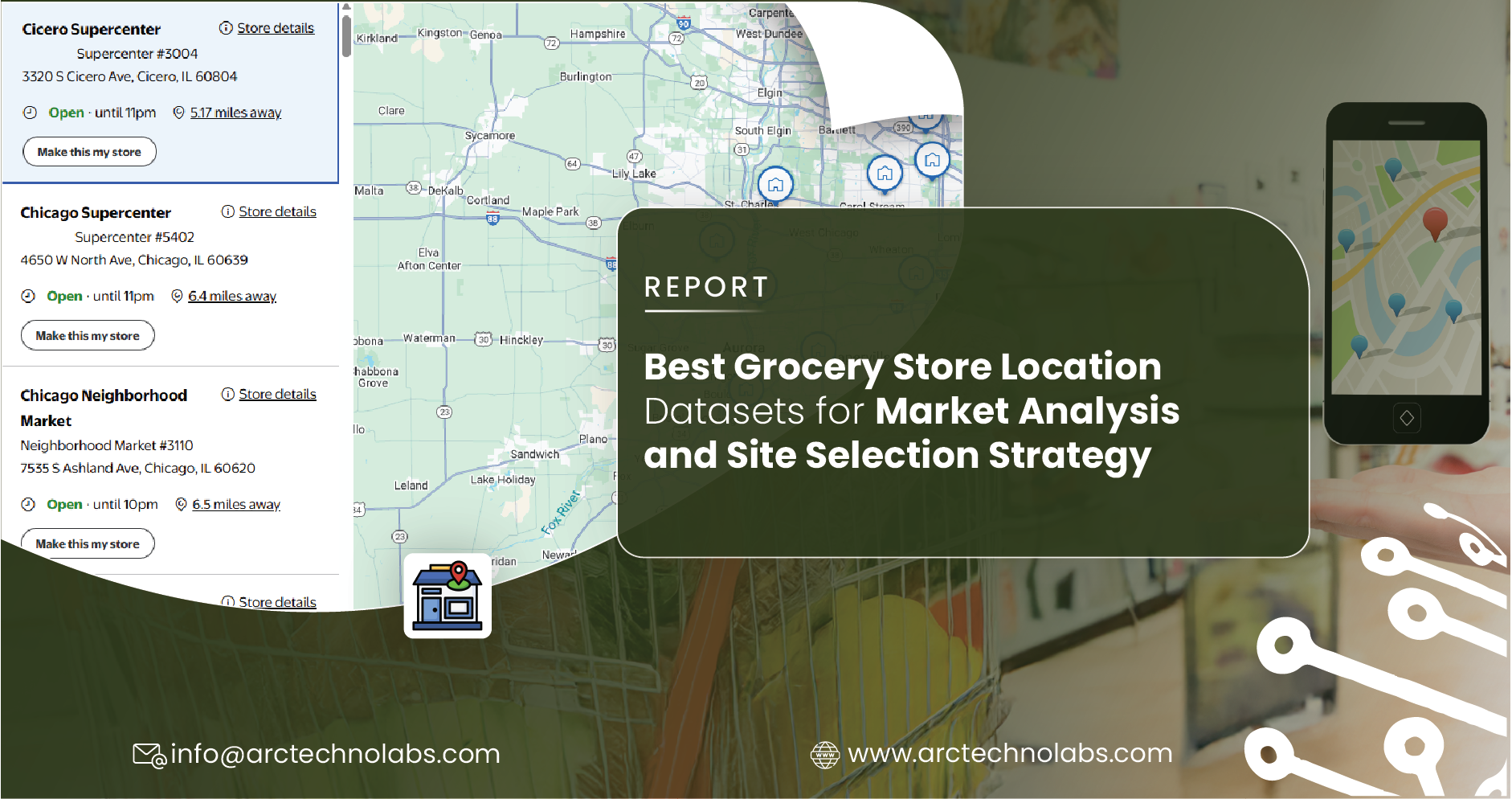
Introduction
In the era of data-driven decision-making, the importance of accurate and comprehensive Grocery Store Location Datasets cannot be overstated. Businesses seeking optimal site selection, market penetration, or competitive pricing analysis rely heavily on high-quality, geo-tagged data.
With the rise of omnichannel retail, hyperlocal marketing, and Q-commerce models, the demand for Grocery and Supermarket Datasets has increased dramatically. These datasets offer actionable insights that support critical business operations such as new store planning, logistics optimization, pricing strategy, and customer targeting.
This report by ArcTechnolabs explores the Best Grocery Store Location Datasets available and how they contribute to successful market analysis and site selection strategies.
Growth of Grocery Location Data Usage in Business Intelligence (2020–2025)
Year | % of Grocery Retailers Using Location Intelligence | Annual Spend on Grocery Location Datasets (Global, in $M) | % Retailers Reporting Improved Site Selection Accuracy |
---|---|---|---|
2020 | 41% | $980M | 39% |
2021 | 52% | $1.32B | 47% |
2022 | 63% | $1.76B | 55% |
2023 | 71% | $2.24B | 62% |
2024 | 78% | $2.82B | 68% |
2025* | 85% (est.) | $3.44B (est.) | 75% (est.) |
Importance of Grocery Store Location Datasets
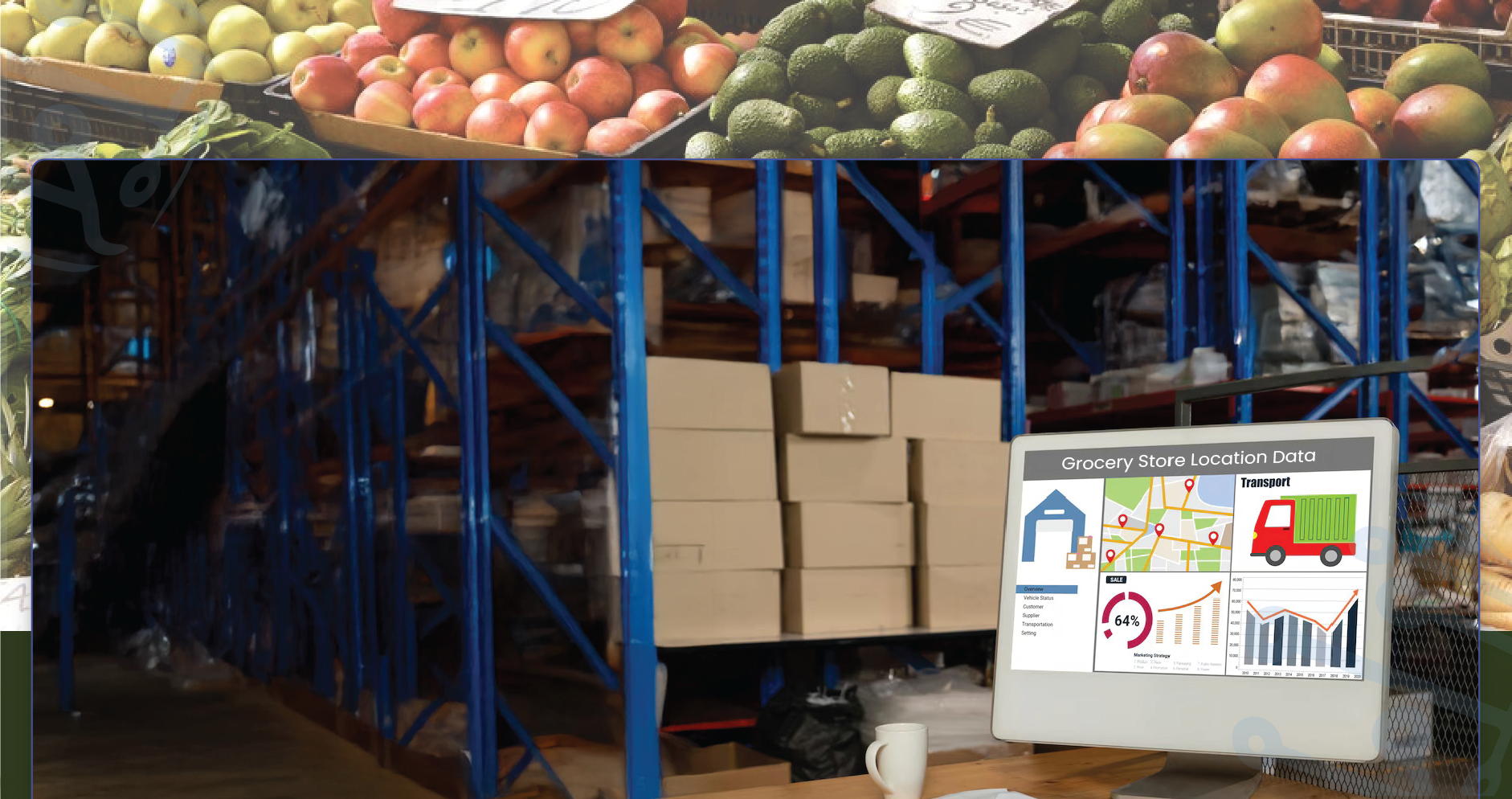
The strategic placement of a grocery store significantly impacts customer footfall, revenue generation, and overall business success. Grocery and Supermarket Datasets offer crucial insights into:
1. Demographics & Regional Demand
Region | Average Household Income (USD) | Population Growth Rate (2020–2025) | Key Consumer Segments |
---|---|---|---|
North America | $68,000 | 2.1% | Urban families, Millennials |
Europe | $62,000 | 1.8% | Seniors, Health-conscious |
Asia-Pacific | $30,000 | 3.5% | Young professionals, Low-income |
Latin America | $15,000 | 2.9% | Working-class, Bargain shoppers |
Insight: Understanding demographics and regional demand is crucial for determining the optimal location for grocery stores, ensuring they cater to the right audience. Best Grocery Store Location Datasets provide this information for precise targeting.
2. Competitor Proximity
Competitor Distance (miles) | Number of Competing Stores | Market Saturation (%) | Retailer Share (%) |
---|---|---|---|
0-1 | 3-5 | 15% | 20% |
1-3 | 6-8 | 30% | 25% |
3-5 | 9-12 | 40% | 30% |
5+ | 12+ | 50% | 25% |
Insight: Competitor proximity is essential for defining a store's competitive advantage. A high concentration of competitors may indicate oversaturation, while a lack of competition could signal an underserved market. Grocery Store Location Datasets help identify these opportunities.
3. Consumer Traffic Flow
Region | Peak Shopping Hours (Avg. Visits per Hour) | Foot Traffic (%) Growth (2020–2025) | Most Visited Store Type |
---|---|---|---|
North America | 250 | 15% | Supermarkets |
Europe | 200 | 12% | Discount stores |
Asia-Pacific | 180 | 18% | Hypermarkets |
Latin America | 150 | 20% | Local grocery chains |
Insight: Consumer traffic flow data provides key insights into the best times for grocery store operations and locations with the highest foot traffic. Using a Grocery store location details scraper, businesses can extract this valuable data.
4. Pricing Strategy
Year | Average Grocery Price Change (%) | Top Price Competitive Regions | Price Sensitivity |
---|---|---|---|
2020 | +2.4% | U.S., Western Europe | Medium |
2021 | +3.1% | Canada, Eastern Europe | High |
2022 | +2.9% | Southeast Asia, Latin America | Medium |
2023 | +3.3% | U.S., Australia | High |
2024 | +4.0% | Western Europe, South Africa | High |
2025* | +4.5% (est.) | Global | High (est.) |
Insight:Pricing strategy heavily influences consumer choices and can be optimized by analyzing Web Scraping Grocery Prices. These insights can be obtained from Grocery & Supermarket Datasets.
5. Store Clustering and Saturation
Region | Number of Grocery Stores per 1000 People | Market Saturation (%) | Potential for New Stores |
---|---|---|---|
North America | 2.5 | 65% | Low |
Europe | 3.2 | 70% | Medium |
Asia-Pacific | 1.2 | 50% | High |
Latin America | 0.8 | 40% | High |
Insight:Store clustering and saturation analysis help identify regions with excessive competition or potential growth areas for new grocery stores. By using a Grocery store location details scraper, businesses can find underserved areas ripe for expansion.
By leveraging Grocery Store Location Datasets and Web Scraping Services, businesses can gain a competitive edge in the site selection strategy and market analysis process, optimizing their approach to store placement and pricing.
Types of Grocery Store Location Datasets
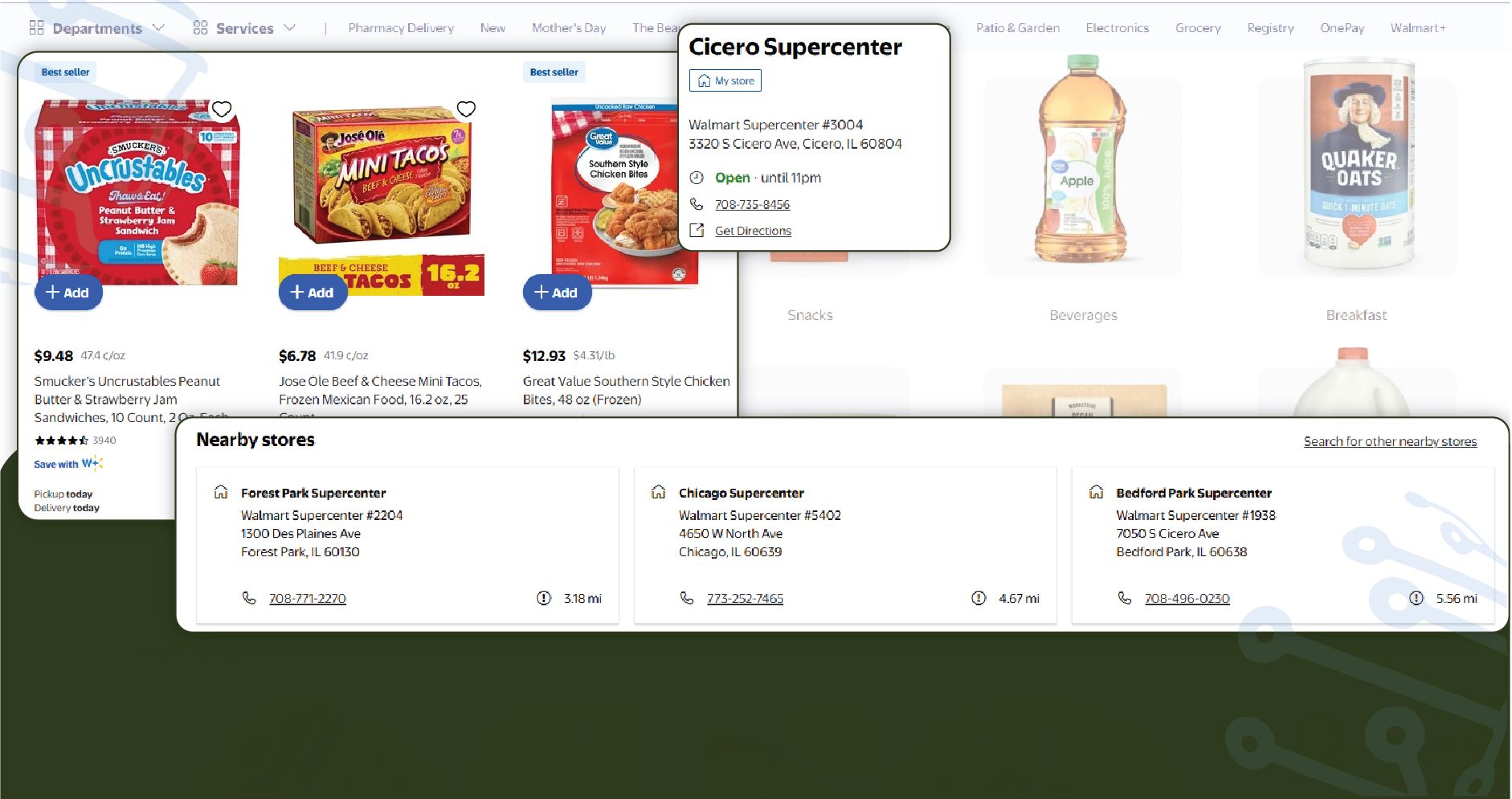
Grocery store location datasets are essential tools for businesses aiming to optimize their site selection and market analysis strategies. These datasets provide valuable insights into various factors such as demographics, foot traffic, competitor proximity, and consumer preferences. By leveraging location data, businesses can identify high-potential areas, assess market saturation, and tailor their pricing strategies effectively. In addition, Web Scraping Services allow real-time data extraction, enabling businesses to stay competitive in dynamic markets. With comprehensive Grocery & Supermarket Datasets, businesses can enhance decision-making, streamline operations, and ultimately boost customer engagement and sales.
Dataset Type | Description |
---|---|
Store Location Coordinates | Latitude, longitude, and address of grocery stores |
Store Attributes Dataset | Store type, size, brand name, amenities available |
Foot Traffic & Visit Frequency | Data from mobile apps and beacons on store visits |
Competitor Mapping Data | Nearby competitors with location and service information |
Consumer Demographics Near Store | Age, income level, family size, and spending patterns of local consumers |
Web Scraping Grocery Prices | Historical and real-time product pricing across various locations |
Mobile App Scraping Services | Data from apps like Instacart, Walmart, Amazon Fresh, and more |
Leading Sources for Grocery Store Location Data (2020–2025)
-01.webp)
Leading sources for grocery store location data provide businesses with accurate, up-to-date information for site selection and market analysis. These sources include government databases, which offer demographic and zoning information, as well as private data providers that specialize in geospatial datasets and foot traffic data. Other sources include market research firms, which compile consumer behavior and competitor location data, and web scraping tools, which extract real-time pricing and location data from grocery store websites. By leveraging these sources, businesses can gain valuable insights into regional demand, competition, and consumer preferences to make informed decisions.
Source | Coverage | Data Points Available | Update Frequency |
---|---|---|---|
Google Maps POI API | Global | Coordinates, name, category, ratings | Real-time |
Yelp & Foursquare APIs | Urban Markets | Business info, reviews, traffic insights | Daily |
ArcGIS & Esri Datasets | U.S. & Europe | Population data, store locations, competitive radius | Weekly |
OpenStreetMap (OSM) | Global | Store tags, amenities, geo-tagged data | Community updated |
Private Data Brokers (e.g., SafeGraph) | U.S. & Canada | Foot traffic, visit duration, geofencing data | Monthly |
Web Scraping Services by ArcTechnolabs | Customizable | Real-time scraping of store listings & prices | On-demand |
Use Cases of Grocery Store Location Data
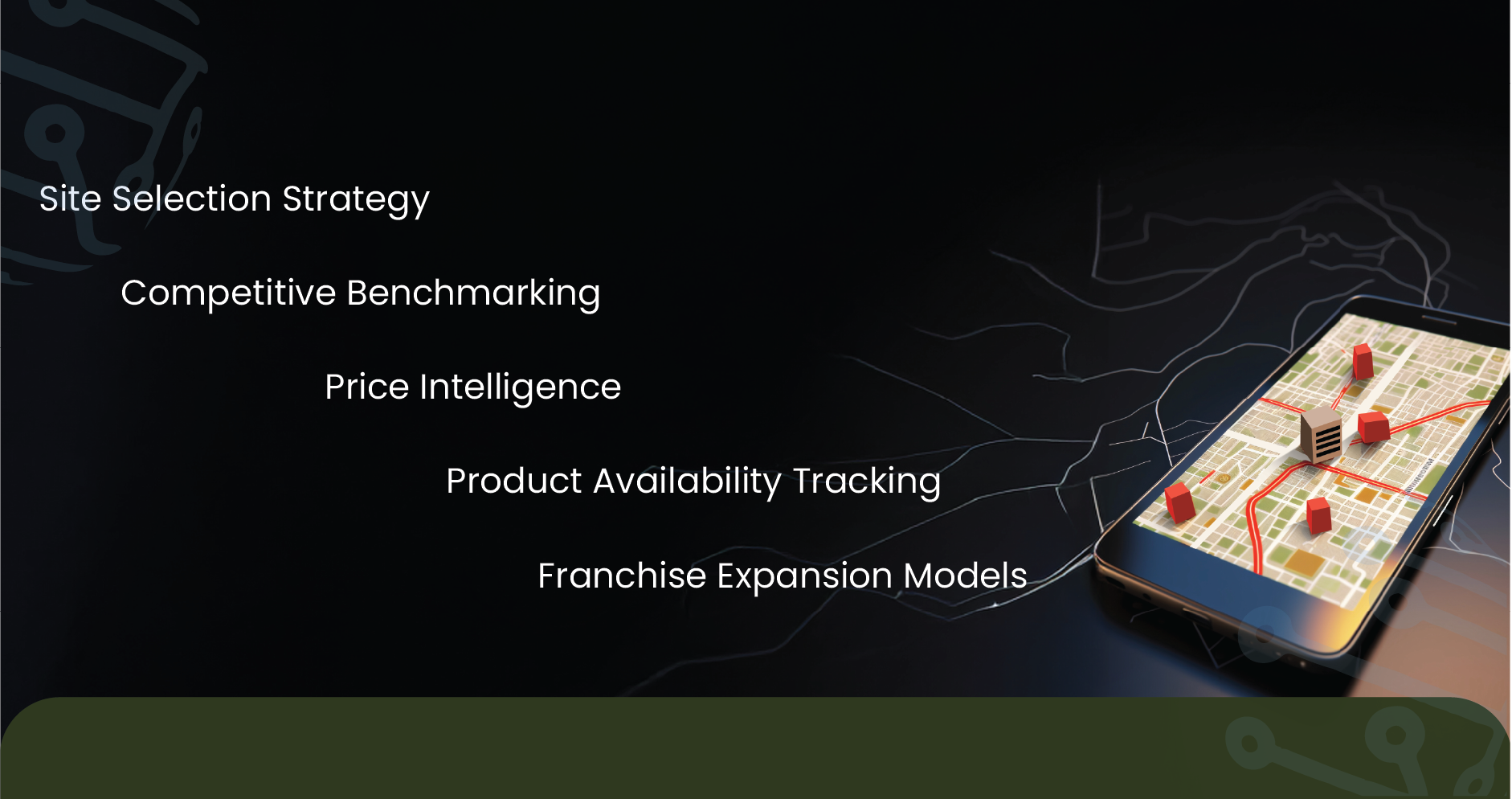
Site Selection Strategy: Identifying Underserved Neighborhoods
Neighborhood | Population | Median Income (USD) | Current Grocery Stores | Market Potential (%) |
---|---|---|---|---|
Downtown Area | 50,000 | $45,000 | 3 | 80% |
Suburban Area | 30,000 | $60,000 | 1 | 75% |
Rural Area | 15,000 | $30,000 | 0 | 90% |
Grocery Store Location Datasets help businesses identify underserved neighborhoods with significant market potential. Using Grocery store location details scraper, businesses can spot areas lacking grocery stores.
Competitive Benchmarking: Mapping Competitors in Radius
Radius (miles) | Competitors in Area | Market Saturation (%) | Retailer Share (%) |
---|---|---|---|
0-1 | 5 | 30% | 25% |
1-3 | 8 | 40% | 35% |
3-5 | 12 | 60% | 45% |
Competitive benchmarking is crucial for understanding competitor distribution within a specific radius. Using Grocery & Supermarket Datasets, businesses can map competitors' locations for informed decisions.
Price Intelligence: Web Scraping Grocery Prices for Optimization
Year | Average Grocery Price Change (%) | Top Competitive Region | Price Sensitivity |
---|---|---|---|
2020 | +2.4% | North America | Medium |
2021 | +3.1% | Europe | High |
2022 | +3.0% | Asia-Pacific | Low |
2023 | +3.8% | Latin America | High |
2024 | +4.2% | U.S., Australia | High |
Web Scraping Grocery Prices allows businesses to track real-time grocery prices and adjust strategies for competitive pricing.
Product Availability Tracking: Monitoring Stock Availability
Region | Average Out-of-Stock Rate (%) | Top Selling Products | Stock Monitoring Frequency |
---|---|---|---|
North America | 5% | Organic Produce, Dairy | Daily |
Europe | 7% | Frozen Foods, Snacks | Weekly |
Asia-Pacific | 3% | Packaged Foods, Beverages | Bi-Weekly |
Latin America | 10% | Canned Goods, Cereals | Monthly |
Product availability tracking via Grocery store location details scraper helps monitor stock levels and optimize supply chains.
Franchise Expansion Models: Selecting High-Potential ZIP Codes
ZIP Code | Population | Average Income | Grocery Stores | Potential for Franchise |
---|---|---|---|---|
90210 | 35,000 | $100,000 | 2 | High |
30301 | 50,000 | $45,000 | 4 | Medium |
33101 | 20,000 | $30,000 | 1 | High |
Insight:Franchise expansion models utilize Grocery & Supermarket Datasets to select high-potential ZIP codes with significant market opportunities for new locations.
These use cases showcase how businesses can leverage Best Grocery Store Location Datasets and Web Scraping Services to drive strategic decisions in site selection, pricing optimization, and market analysis.
Top 50 Best Grocery Store Datasets (2025 Edition)
# | Dataset Name | Data Provider | Region |
---|---|---|---|
1 | Google Maps Grocery POI Dataset | Global | |
2 | Walmart Store Listings Scraper | ArcTechnolabs | U.S. |
3 | Instacart Store Availability Scraper | ArcTechnolabs | U.S./Canada |
4 | Amazon Fresh Location Dataset | Amazon | U.S. |
5 | Kroger Location Intelligence Data | SafeGraph | U.S. |
6 | OpenStreetMap Grocery Tags Dataset | OSM | Global |
7 | Yelp Grocery Business Listings API | Yelp | Global |
8–50 | [Customized Scraped Datasets via ArcTechnolabs] | Tailored | Global |
Scrape grocery store location data with ArcTechnolabs to access curated, geo-tagged, and updated datasets for every requirement.
Market Trends (2020–2025): Datasets Demand Surge
Year | Global Demand for Location Datasets (in $M) | % Growth YoY |
---|---|---|
2020 | 1,050 | — |
2021 | 1,390 | 32.4% |
2022 | 1,870 | 34.5% |
2023 | 2,350 | 25.6% |
2024 | 2,970 | 26.3% |
2025* | 3,680 (est.) | 23.9% |
The demand for Grocery store location details scraper tools and Grocery and supermarket location datasets has seen exponential growth post-COVID due to the boom in hyperlocal commerce and Q-commerce models.
ArcTechnolabs’ Grocery Dataset Solutions
ArcTechnolabs offers specialized Web Scraping Services to collect:
- Store coordinates, business hours, and contact details
- Real-time pricing & availability data from leading online grocery platforms
- Competitor mapping with distance & density insights
- Consumer reviews and sentiment analytics
We also support Web Scraping API Services and Mobile App Scraping Services for platforms like Walmart, BigBasket, Instacart, and Amazon Fresh.
Conclusion
Accessing the Best Grocery Store Location Datasets is critical for any retailer, investor, or franchise operator planning expansion. By integrating Grocery & Supermarket Datasets with location intelligence, businesses can optimize resource allocation, avoid saturation, and identify untapped opportunities.
ArcTechnolabs empowers enterprises to scrape grocery store location data efficiently with tailored scraping pipelines and robust APIs. Contact us to know more!